Authorisation
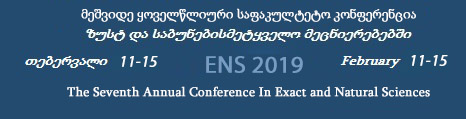
On Intelligent Character Recognition for Georgian Handwriting
Author: Magda TsintsadzeCo-authors: M.Khachidze, M.Archuadze
Keywords: Machine learning, data processing, OCR, ICR
Annotation:
Data processing is an integral part of everyday life and is one of the main problems of modern research; The problem of handwriting computational processing remains an unsolved problem for many languages and, in particular, for Georgian (Khachidze et al., 2017). Most of the works exist exclusively in handwritten form, and significant labor force is expanded by manually typing such texts in order to save them and convert them into editable form. This problem is especially relevant in office workstations, since manual creation of searchable and editable backups of partially or fully handwritten documents can take a lot of time and resources. As a result, the creation of a highly accurate handwriting recognizer has been a priority for decades. Although significant progress has been made in recognition systems designed to recognize Latin characters (Patel et al., 2012), character sets in many languages, including Georgian, do not yet have recognition models or data sets of acceptable quality. Intelligent Character Recognition (ICR) is an advanced optical character recognition (OCR) system or, more precisely, handwriting recognition software that allows computers to learn fonts and different styles of handwriting input during processing to improve accuracy and level of recognition. Handwriting recognition consists of many stages (pre-processing, segmentation, feature extracting, classification) and in general it can be classified into two types of recognition: On-line and Off-line recognition: Sequential (on-line) classification systems deals with data stream and classify characters using stroke data, such as movement directions, speed, intervals. With the rise in the usage of hand-held devices using touchscreens and similar sensors the need for sequential handwriting recognition (e.g. recorded movement of stylus or fingers using a touchscreen on a tablet) rather than visual (e.g. photocopied documents) has also increased. Utilizing the extra data gathered in the writing process (such as directions of the stroke) the sequential handwriting recognition has been able to offer higher accuracy while using a smaller number of samples for training. Non-Sequential (off-line) classification is connected with image processing directly and is considered to be rather difficult than its congener. One of the tasks of implementing Handwritten recognition from images is to create a large data set of handwritten characters, including the handwriting of many people. Selecting a recognition model based on machine learning is of high importance as well. Options include various artificial neural network architectures, including VGG, ResNet, Inception, and other approaches, such as support vector machines. In the article the issue of Georgian handwriting ICR model is considered and Self-Normalizing Convolutional Neural Networks (CNN) application is proposed for the best performance.